The Future of AI in Financial Trading: What to Expect
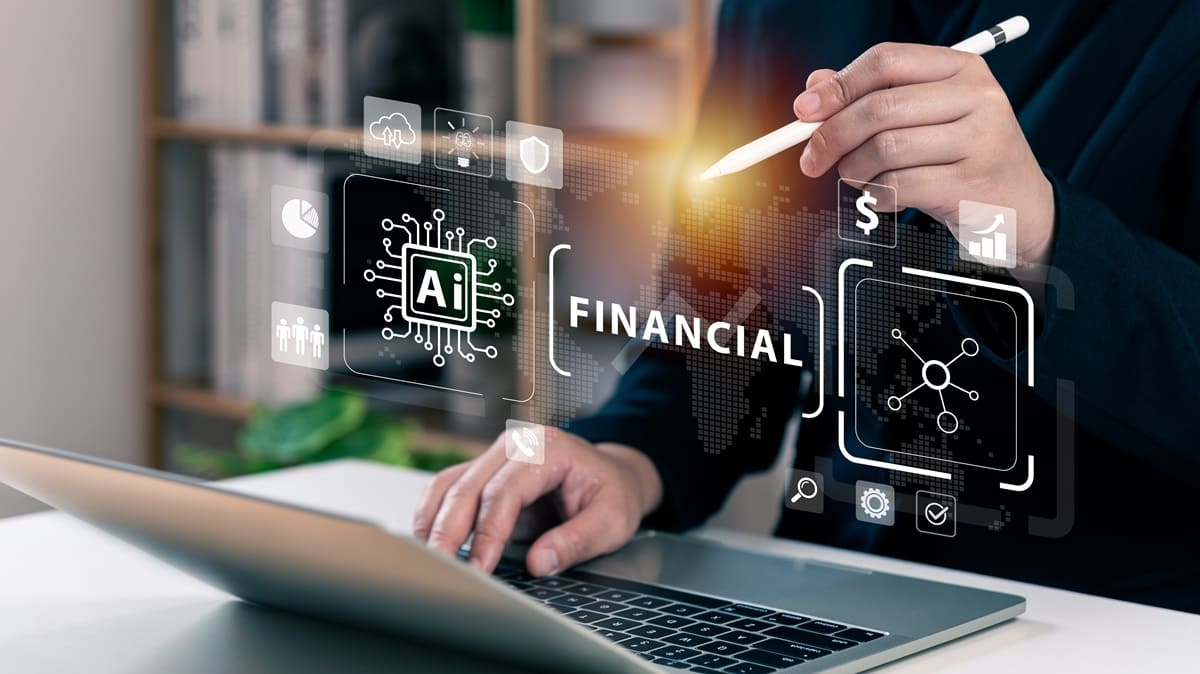
Artificial Intelligence (AI) is not just a buzzword anymore; it’s revolutionizing industries, including the financial markets. In financial trading, AI has become a game-changer, providing tools to analyze large data sets, predict market trends, and execute trades at speeds no human can match. But what does the future hold for AI in this high-stakes world? Let’s explore the advancements and what we can expect in the coming years.
The Evolution of AI in Financial Markets
Early Adoption of AI in Financial Trading
The use of AI in financial markets first emerged in the early 2000s with the development of algorithmic trading. During this time, basic AI-driven algorithms were designed to automate specific trading tasks that were previously done manually. One of the early applications was the ability to break down large orders into smaller chunks to avoid impacting the market prices significantly. These systems were rudimentary by today’s standards, but they were revolutionary for the time. They allowed traders to automate repetitive tasks and reduce human error, paving the way for more sophisticated AI tools in the years to come.
The early adoption of AI was primarily focused on efficiency and speed. Traders and financial institutions began to realize the potential of AI for handling large volumes of data and executing trades at a much faster pace than human traders. This period marked the foundation of what would become the modern era of AI in financial trading. Despite the limitations of early AI systems, their introduction brought significant advantages, such as improved accuracy in order execution and the ability to trade in fractions of seconds, which was impossible before the digital revolution.
Modern-Day AI in Trading
In today’s financial markets, AI has evolved significantly beyond simple algorithms. Modern AI systems incorporate advanced machine learning techniques that allow them to not only execute trades but also analyze market trends, predict future price movements, and even adapt strategies in real-time. These AI systems leverage vast amounts of data—from historical market data to current news, social media sentiment, and financial reports—to make informed trading decisions. Machine learning models can identify complex patterns in data that may not be visible to human traders, giving institutions that use AI a competitive edge in the market.
Automated trading systems have become commonplace, allowing traders to set predefined rules for their trading strategies, which are then executed automatically by AI without human intervention. This shift has brought about a new era of high-speed, high-frequency trading, where AI can make thousands of trades within seconds. However, this advancement is not without its challenges. While AI offers enhanced speed and accuracy, it also introduces risks, such as over-reliance on algorithms and potential system failures. Thus, while modern AI systems offer remarkable capabilities, they also demand careful oversight to avoid catastrophic losses.
How AI is Currently Used in Financial Trading
AI-Powered Algorithms for High-Frequency Trading
High-frequency trading (HFT) is one of the most prevalent applications of AI in financial markets today. HFT involves the use of AI algorithms to execute a large volume of orders at extremely high speeds, often in fractions of a second. This method relies heavily on the precision and speed of AI to identify small price discrepancies across markets and capitalize on them before other traders have the opportunity to react.
AI-powered algorithms are particularly effective in HFT because they can:
- Monitor multiple markets simultaneously.
- Analyze real-time data streams to detect trading opportunities.
- Execute trades faster than any human trader could possibly achieve.
These systems are designed to spot fleeting opportunities that only exist for milliseconds and exploit them for profit. However, the reliance on AI in HFT also has its drawbacks. These systems can sometimes amplify market volatility, especially during periods of instability, leading to large-scale price swings.
AI for Market Analysis and Prediction
Beyond executing trades, AI has become an invaluable tool for market analysis and prediction. Traditional methods of market analysis often involved laborious processes where analysts would manually sift through financial data, news reports, and economic indicators. AI systems, however, can process vast amounts of data in a fraction of the time, allowing for more accurate and timely market predictions.
For example, AI systems can:
- Analyze news articles, social media posts, and company reports to assess market sentiment.
- Use machine learning to identify patterns in historical market data.
- Predict price movements based on real-time data inputs.
These systems provide traders with insights that would be impossible to gather manually, allowing them to make more informed decisions. Moreover, AI can continuously learn and improve its predictive models, making them more accurate over time. Despite these benefits, it’s important to remember that predictions are not always foolproof. Markets can be influenced by a range of unpredictable factors, making it essential for traders to use AI insights alongside human judgment.
AI in Risk Management
Risk management is another area where AI has made significant strides. Traditional risk management strategies rely on historical data and market analysis conducted by human analysts. While effective to a certain extent, this approach has limitations, especially in a fast-moving market. AI, on the other hand, can enhance risk management by analyzing real-time data and identifying risks as they emerge, rather than reacting to them after the fact.
AI in risk management can:
- Monitor market conditions continuously for signs of increased risk.
- Detect anomalies and potential threats that human analysts might miss.
- Suggest proactive measures to mitigate risks before they escalate.
These capabilities allow firms to protect their portfolios more effectively and make better-informed decisions about when to enter or exit the market. Additionally, AI can help in stress-testing portfolios under various market conditions, providing insights into how different scenarios could affect performance. However, it’s essential to balance AI-driven risk management with human oversight to ensure that potential issues, such as algorithmic biases or system malfunctions, are adequately addressed.
AI and Machine Learning Techniques Impacting Trading
Reinforcement Learning in Trading
Reinforcement learning (RL) is a cutting-edge AI technique that is rapidly gaining traction in the world of financial trading. Unlike traditional machine learning models, which rely on historical data to make predictions, reinforcement learning agents learn by interacting with the environment. In the context of trading, this means that RL systems “experiment” with different strategies, continuously refining and improving their approach based on the feedback they receive from the market. For example, an RL system may test a certain buying strategy, observe the market’s reaction, and then adjust its strategy accordingly. Over time, these systems become better at optimizing trading decisions, which can lead to more profitable outcomes.
One of the main advantages of reinforcement learning in trading is its adaptability. Markets are dynamic and ever-changing, with numerous variables impacting price movements. An RL agent can learn to adapt to these fluctuations, making it especially effective for developing strategies that need to account for complex market behavior. The continuous learning process allows reinforcement learning systems to optimize their performance over time, often outperforming static, rule-based trading algorithms. This ability to learn and improve autonomously makes reinforcement learning one of the most promising techniques for the future of AI in trading.
Natural Language Processing (NLP) for Market Sentiment
Natural Language Processing (NLP) has become a critical tool for understanding market sentiment, an essential component of modern trading strategies. Market sentiment refers to the overall attitude of investors toward a particular asset or the market as a whole. NLP systems can analyze vast amounts of unstructured text data from sources such as news reports, social media, earnings calls, and financial statements. By processing this data, NLP models can gauge whether the sentiment is positive, negative, or neutral and then correlate this sentiment with future price movements.
For instance, if a company is involved in a scandal and there is widespread negative media coverage, an NLP model might detect this negative sentiment and predict a drop in the company’s stock price. On the other hand, positive news about a company’s growth prospects could indicate that the stock price will rise. By quickly processing and analyzing massive amounts of text data that would be overwhelming for a human analyst to parse through, NLP provides traders with valuable insights that help them stay ahead of the market. This ability to read and interpret natural language is a powerful tool for anticipating market trends and making informed trading decisions.
AI Technique | Function | Impact on Trading | Example Use Case |
---|---|---|---|
Reinforcement Learning | Learns through interaction with the market environment. | Continuously improves trading strategies based on feedback. | Developing adaptive, self-optimizing trading strategies. |
Natural Language Processing (NLP) | Analyzes text data to understand market sentiment. | Predicts market movements based on news and social media. | Detecting sentiment shifts around a specific company stock. |
Benefits of AI in Financial Trading
Enhanced Speed and Efficiency
One of the most transformative benefits of AI in financial trading is the enhancement of speed and efficiency. Traditional manual trading often involves long, time-consuming processes—evaluating market conditions, analyzing data, and executing trades. Human traders, regardless of their expertise, simply cannot match the speed at which AI-driven systems operate. AI algorithms can execute trades in milliseconds, processing complex data sets far faster than any human could. This incredible speed is particularly beneficial in high-frequency trading (HFT), where small, quick price changes are exploited for profit. With AI, traders can capitalize on opportunities that last for mere seconds, ensuring that no valuable trades are missed.
This speed also results in greater efficiency, as AI systems can automate much of the trading process, reducing the need for human intervention in repetitive or mundane tasks. For example, once an AI-driven system is programmed with a particular strategy, it can execute that strategy autonomously without requiring constant monitoring from the trader. This allows human traders to focus on higher-level strategy and decision-making rather than getting bogged down in the minutiae of executing individual trades. Overall, AI’s ability to streamline the trading process means more trades can be executed with greater precision and less time, helping traders maximize their returns.
Improved Decision-Making
AI also offers significant improvements in decision-making by providing traders with insights that are not easily discernible through traditional analysis methods. AI systems are designed to process and analyze vast amounts of data—far beyond what a human trader could handle. These systems can quickly identify patterns and trends in the market, using this information to predict future movements and guide trading decisions. For example, AI can process historical price data alongside real-time market conditions to suggest whether a trader should buy, hold, or sell a particular asset.
Moreover, AI-driven systems continuously learn from new data, improving their predictive models over time. This means that as the market evolves, AI can adjust its strategies accordingly, helping traders stay ahead of the curve. By offering a deeper, more data-driven approach to trading, AI enhances decision-making, allowing traders to act on more accurate and timely information. Whether it’s detecting early signs of a market downturn or identifying a new investment opportunity, AI can provide traders with actionable insights that lead to better outcomes.