How AI is Changing the Landscape of Trading
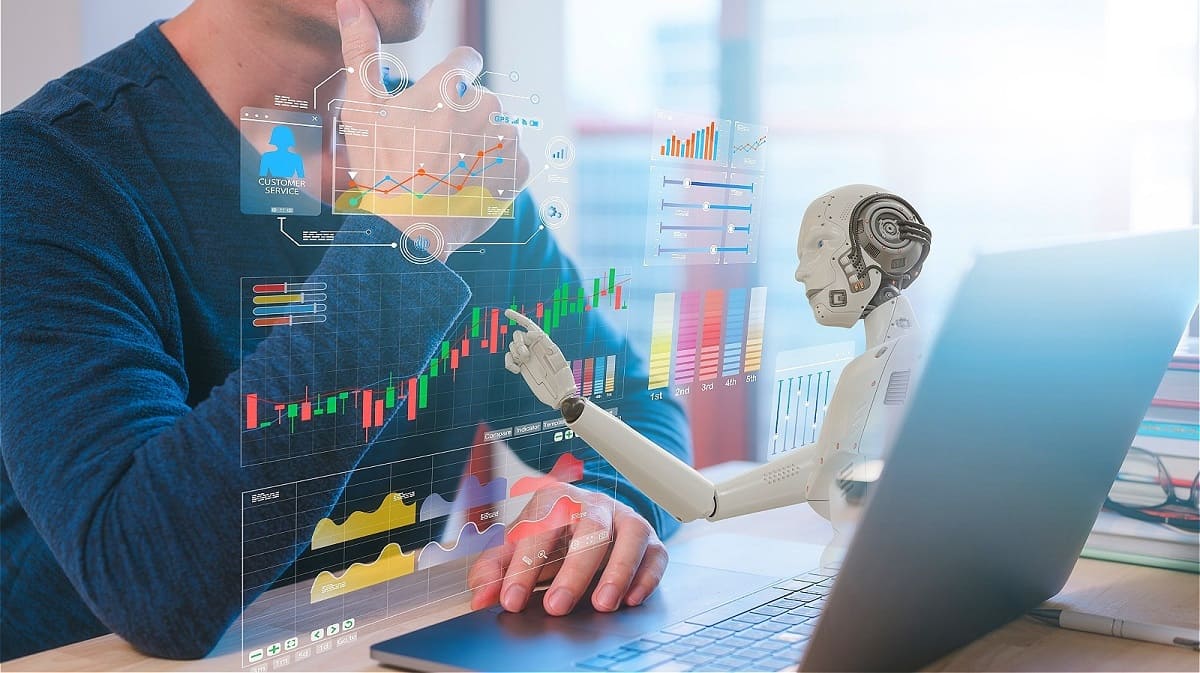
Artificial intelligence (AI) has become a transformative force across industries, from healthcare to transportation. But perhaps one of the most fascinating and fast-growing areas of AI’s influence is in the world of trading. With its ability to process vast amounts of data, make complex calculations, and predict market trends, AI is reshaping how trades are executed, managed, and profited from.
The Rise of AI in Financial Markets
Over the last decade, artificial intelligence (AI) has increasingly become integrated into financial markets, bringing about significant changes that have redefined trading practices. What once began with relatively simple automated trading systems has evolved into sophisticated AI-driven platforms that can process vast amounts of data and execute trades with incredible precision. One of the most critical milestones in this development was the rise of machine learning in the early 2010s. Machine learning enabled AI to “learn” from historical data, recognizing patterns and improving its performance over time without explicit programming. As AI became more intelligent, its role in financial markets expanded beyond automation, moving into areas such as predictive analytics and decision-making. This rise of AI has transformed trading strategies and improved market efficiency, as AI can analyze vast amounts of data quickly and adapt to changing market conditions.
AI’s ability to process large datasets has also led to the rise of more accurate predictive models. Predictive analytics has allowed traders and financial institutions to forecast market trends with greater precision, improving their ability to capitalize on opportunities or mitigate risks. Additionally, AI has opened up new opportunities for algorithmic and high-frequency trading. By using machine learning algorithms, AI can react to market shifts in microseconds, giving traders an edge in rapidly fluctuating markets. These advances have made AI indispensable in today’s financial landscape, with hedge funds, investment banks, and even retail traders relying on AI to enhance their trading strategies and decision-making processes. The integration of AI in financial markets is not just a passing trend but a fundamental shift in how trading will continue to evolve in the future.
AI in Trading
AI in trading refers to the use of artificial intelligence technologies to automate, enhance, or entirely take over various trading processes. At its core, AI helps traders make more informed decisions by analyzing vast datasets, identifying patterns, and predicting future market trends. Unlike traditional trading methods that rely on human analysis and manual execution, AI systems can sift through an enormous amount of data, such as historical prices, economic indicators, and even news sentiment, all in real-time. This capability enables AI to manage trades far more efficiently than a human could, allowing it to execute trades with precision, speed, and accuracy.
The most common AI technologies used in trading include machine learning, natural language processing (NLP), and deep learning algorithms. Machine learning helps AI systems recognize patterns in market data, enabling them to make predictions and continuously improve their performance over time. NLP, on the other hand, allows AI to analyze text data, such as financial reports, news articles, and even social media sentiment, to gain insights into market movements. Deep learning, a subset of machine learning, takes this a step further by enabling AI systems to analyze unstructured data, such as images and voice recordings, which can also affect market trends. These AI technologies work together to enhance trading processes, ultimately making them more efficient, profitable, and reliable.
Advantages of AI in Trading
AI offers several key advantages that make it an appealing tool for both institutional and retail traders alike. Here are some of the most significant benefits:
- Enhanced Data Analysis: AI can process massive amounts of data in seconds, a task that would take human analysts days or even weeks to complete. It can analyze various data sources such as historical prices, trading volumes, economic reports, and even market sentiment from news articles or social media posts. By processing and analyzing this data rapidly, AI provides traders with actionable insights that help them identify trends and make informed decisions.
- Speed and Accuracy: AI can execute trades at lightning speed and with precision. Automated AI trading systems can identify opportunities in the market and execute trades in microseconds, much faster than any human trader could. This speed is particularly valuable in high-frequency trading (HFT), where every millisecond counts. AI’s accuracy in executing trades also reduces the risk of costly human errors, ensuring that trades are conducted exactly as programmed.
- Emotion-Free Trading: Human emotions such as fear and greed can often cloud judgment, leading to irrational trading decisions. AI, however, operates purely based on data and predetermined rules, eliminating emotional bias. This results in more rational and consistent trading strategies, especially in volatile markets where emotions can run high. By sticking to data-driven decisions, AI systems can often achieve better long-term performance than emotionally-driven human traders.
AI-Powered Trading Systems
AI-powered trading systems are advanced software platforms that use artificial intelligence to conduct trades automatically. These systems are designed to analyze vast amounts of market data, such as historical price movements, economic indicators, and real-time news, and then use this information to make informed trading decisions. Unlike traditional trading methods, which often require a human trader to interpret data and execute trades manually, AI-powered systems can make decisions and execute trades at an incredibly fast pace, without human intervention. These systems are capable of learning from historical market patterns, adjusting their strategies based on new data, and even predicting future market trends using sophisticated algorithms. This ability to analyze data, learn, and adapt makes AI-powered trading systems particularly effective in volatile and fast-moving markets.
A great example of the success of AI-powered trading systems is Renaissance Technologies, a highly successful hedge fund known for its quantitative and AI-driven approach. Renaissance Technologies uses AI to analyze complex financial data and execute trades with a high degree of accuracy. Their AI-powered systems have consistently outperformed the market, proving that AI can be a powerful tool in achieving profitability. The success of Renaissance Technologies has inspired other financial institutions and individual traders to adopt AI-driven trading platforms, recognizing the immense potential AI offers in terms of accuracy, speed, and adaptability in trading.
Aspect | AI-Powered Trading Systems | Traditional Trading Methods | Key Advantage of AI |
---|---|---|---|
Data Analysis Speed | Extremely fast | Slow and manual | Speed |
Decision Making | Automated, based on AI models | Manual, human-driven | Automation |
Adaptability | Learns and adjusts over time | Static without AI intervention | Adaptability |
Execution Accuracy | High precision | Prone to human error | Accuracy |
Algorithmic Trading and AI
Algorithmic trading, often referred to as “algo-trading,” involves using computer algorithms to automate trading decisions based on pre-set criteria such as timing, price, or volume. Traditional algorithmic trading systems have been in use for decades, but the introduction of AI has significantly enhanced their capabilities. AI algorithms are able to analyze massive amounts of data, identify complex market patterns, and make decisions in real-time. This allows AI-enhanced algorithmic trading systems to be more adaptive to sudden market changes and fluctuations, leading to faster, more accurate, and more profitable trades. The main advantage of AI in algorithmic trading is its ability to process information and make adjustments much faster than a human trader ever could, allowing it to react to changing market conditions in fractions of a second.
AI is particularly effective in optimizing algorithmic strategies, meaning that AI-powered systems can learn from historical data and refine their performance over time. This results in improved profitability, as the AI becomes better at identifying profitable trading opportunities while minimizing risk. Today, many of the largest algorithmic trading firms rely heavily on AI to enhance their trading strategies and stay competitive. Whether it’s high-frequency trading, where speed is crucial, or more long-term trading strategies that rely on predictive analytics, AI plays a critical role in improving the efficiency and effectiveness of algorithmic trading.
Machine Learning in Trading
Machine learning, a subset of AI, has become one of the most important technologies in modern trading due to its ability to “learn” from data. Unlike traditional algorithms, which operate according to predefined rules, machine learning models can adapt and improve their performance over time by analyzing historical data and adjusting their strategies accordingly. In trading, machine learning is used to identify patterns in market data, such as price movements or trading volumes, which can then be used to predict future trends. For example, a machine learning model might be trained on years of stock price data to recognize patterns that precede significant price movements, allowing the system to anticipate and act on market opportunities before they occur.
There are several types of machine learning models used in trading, each with a specific application. Supervised learning models are trained on labeled data, where the system is provided with historical inputs and corresponding outputs, such as past stock prices and subsequent market movements. The model then learns to predict future outcomes based on this data. Unsupervised learning models, on the other hand, do not require labeled data and are used to detect hidden patterns or anomalies in the data. This can be particularly useful in identifying unusual trading activity that may signal an upcoming market event. Reinforcement learning is another machine learning model where the system learns by interacting with its environment and receiving feedback in the form of rewards or penalties. In trading, reinforcement learning models can be used to develop strategies that maximize long-term rewards by continuously adjusting to market changes.